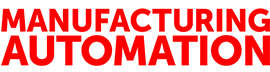
Learning 4.0: Bin picking – the holy grail of automation
January 25, 2022
By Yarek Niedbala
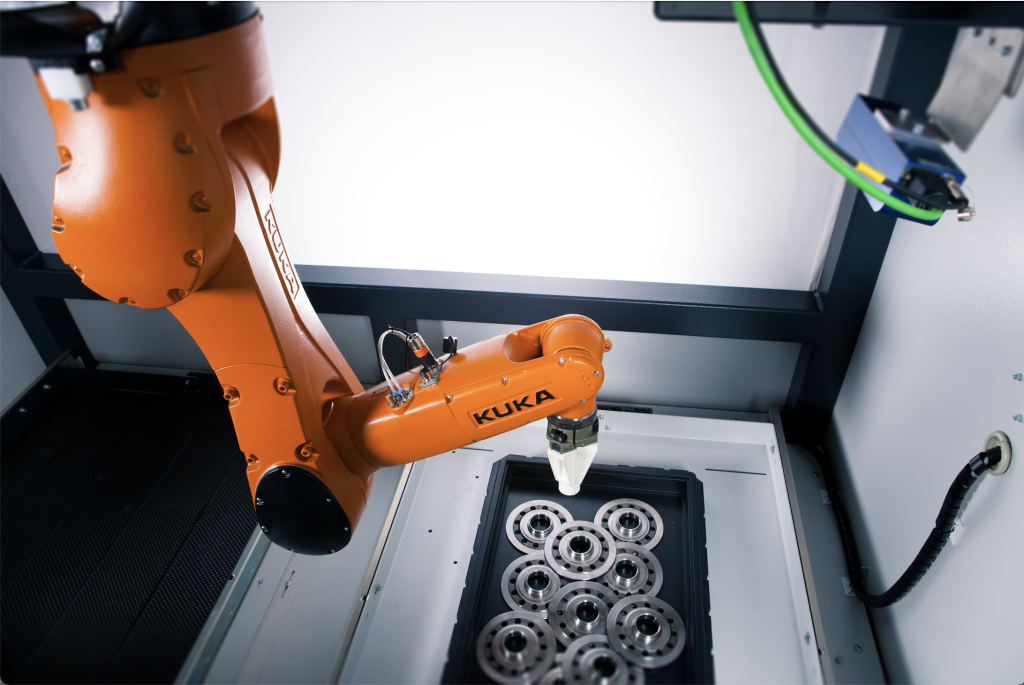
To humans, picking out randomly situated items from a bin is a simple act that most individuals could perform blindfolded, but for a robot, the task is quite complex. Robots cannot be programmed to bin pick, per se. The task requires advanced vision technology coupled with machine learning through artificial intelligence (AI) that involves high levels of computer processing speed and power. By incorporating these breakthrough technologies into one system, robots can now perform what those in the automation world once referred to as the “holy grail of automation,” otherwise known as bin picking.
Working with Roboception GmbH, a pioneer of 3D sensor technology, Kuka Robotics developed a cost-effective system that achieves accurate robotic bin picking. Together, the two companies perfected the system that successfully blends the physical (robot) with the digital (AI). This allowed users to quickly configure and deploy the automation through a user-friendly web Graphical User Interface (GUI) without the need for special skill sets.
In essence, the system allows the robot to identify which parts it can pick from a bin, and which are not oriented in a reachable position, or are obscured by another part. For those parts that are accessible but not in a perfect pick position, the robot makes quick adjustments. Model tooling enables quick identification of possible interference with the bin and allows the robot to determine if parts near the wall are pickable, therefore avoiding any collisions with a bin wall.
3D stereo camera systems and applied AI are the keys to successful automated bin picking. The camera recognizes parts – not just their location, but also their orientation – as it scans the entire contents of a bin and relays the data to the software. In the past, the processing of such vision data took a long time. So, systems had to use two cameras and two bins. While the robot figured out which part could be picked in one bin, the camera system processed the data from parts in the second bin. The Kuka system removes those limits by processing data faster with limited delay and using only one camera.
In this case, the physical aspect of the system is the robot, and the digital aspect is software that models the parts in the bin, along with AI that selects a part and determines how the robot needs to position itself for the extraction. For the Kuka system, the 3D stereo camera/vision system captures a part image and transfers it to the Roboception software, which then uses the images to extract data representing viable parts that the robot can pick. From the image, the software rates which part is in the optimal pick position or relatively close to it, then sends decisions to the robot. While some of the robot’s moves are preprogrammed/taught, the vision system and AI generate and dictate other required movements – for the actual part picking – on the fly. AI automates the part detection and classification process, or what is known as parameter tuning. However, the task requires fast and powerful computing resources and special algorithms.
Roboception applies machine learning to execute simulations of various scenarios, tests them in the virtual world, then develops what is called a “detection template” that works best in all possible conditions. These scenarios can involve various lighting conditions and bin sizes along with bin filling levels and orientations, in addition to many others.
Instead of determining all possible configurations – a task that integrators had to perform onsite – the template is generated offline using design data to train the robot. In this case, the machine learning is no longer dependent on extremely large ground-truth datasets but can be driven through design data – such as CAD models – in a simulation environment. This is considered a key breakthrough in automated bin picking.
One application for such highly efficient model-driven bin picking involves bolts or fasteners typically found in the automotive or aerospace sectors. In most instances, some type of feeder system like a bowl feeder is used. This often jams up, causing production stoppages. To prevent these bottlenecks, automated bin picking can eliminate the need for such feeder systems and provide greater reliability while helping to reduce overall production downtime.
To demonstrate these automation applications to small- and medium-sized enterprises (SMEs) and other companies, Kuka often partners with the Barrett Centre for Technology Innovation (Barrett CTI) at Humber College Institute of Technology and Advanced Learning in Toronto, Ont. Kuka is a founding partner of Humber’s Advanced Manufacturing Skills Consortium, which brings together interdisciplinary teams of faculty, students and industry experts to solve complex, real-world problems.
The Barrett CTI acts as an outsourced research and development department for companies such as Kuka, providing a supportive, low-risk environment for collaboration with students and faculty. These collaborations experiment with leading-edge technologies, transforming ideas into operational efficiencies and marketable products and services. This is the industry working with educators to fill the skills gap, enabling more technological breakthroughs in the future.
Yarek Niedbala is the vice-president of sales for Kuka Robotics Canada, a provider of intelligent automation solutions including industrial, collaborative and mobile robots, linear tracks, positioners, machine vision, AGVs and Industry 4.0 technologies.
Advertisement
- Blackline Safety announces $2 million investment to enhance manufacturing capacity
- Hannover Messe 2022 to take place in the beginning of June