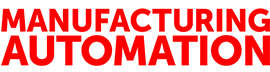
Far-sighted vision: Advancements in artificial intelligence to enhance machine vision adoption
November 22, 2022
By Jack Kazmierski
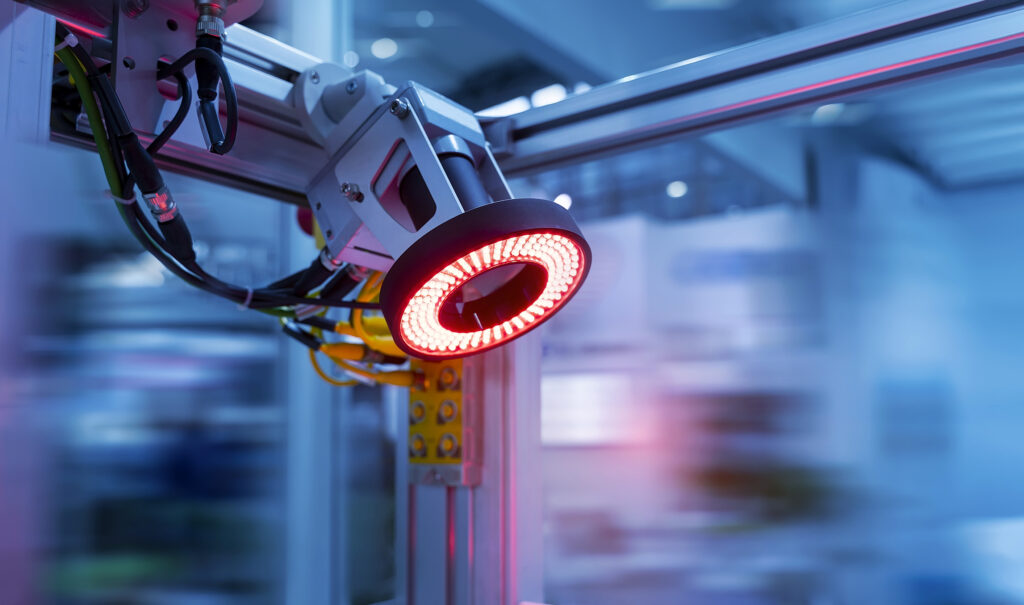
Rising costs, a broken supply chain and a human resources situation that has been complicated by the pandemic have made it a challenge for manufacturers to stay competitive in today’s changing world. In order to limit risk and boost profitability, some are turning to machine vision automation to cope, as well as prepare for tomorrow’s business landscape.
“Staying competitive,” says Donato Montanari, vice-president and general manager of machine vision at Zebra Technologies, “is really about the cost of the product and access to labour.”
Montanari explains that machine vision can help manufacturers tackle these challenges by automating some of the labour-intensive aspects of the manufacturing processes. He offers the example of a parts manufacturer dealing with elevated costs, exacerbated by a shortage of certain parts, having to deal with imperfections or mistakes that result in a defective end product that needs to be discarded because it can no longer be sold.
“This is where inspection and quality control become more and more important,” he says. “With the old approach, quality control was done by a person at the end of the line. Today, the problem is you don’t have access to those people anymore. Those people don’t scale, and that labour is among the most specialized and most expensive in your company, because that’s where the value is.”
The solution could be machine vision, which is scalable, which can be as specialized as necessary, and which once trained can work around the clock and never take a day off.
The importance of quality control, and the importance of track and trace, which are the two predominant applications of machine vision, have become increasingly significant today. “And this is how machine vision can help discrete manufacturing, enabling them with automation,” Montanari explains.
Abhishek Sarkar, lead data scientist at Siemens Advanta Solutions agrees, adding that machine vision can do what the human eye sometimes can’t. “You need very well-trained labour to inspect parts in manufacturing and discrete industries,” he explains. “If you have an untrained eye, you might miss a lot of defective parts, so that’s where AI [Artificial Intelligence] can come into the picture.”
Sarkar notes another advantage machines have over humans. “When it comes to manual inspections,” he explains, “they cannot be done 24/7. A human will only take samples every few minutes or hours, and there’s a high chance they will miss a lot of defective parts. So that’s where machine vision comes into the picture. You can have 24/7 monitoring so that the chances of missing a defective part and figuring it out later on when that part has already gone through a downstream process and it’s too late [is minimized].”
Calculating the cost
As practical as machine vision may be, historically, it’s been expensive and time-consuming to implement. Much of the cost has to do with the need for a machine learning engineer, working with a subject matter expert, to program the system to recognize defects so that the system understands what it’s looking for.
“You need a lot of what we call in the machine learning language, label data,” Sarkar explains. “So, you have to feed the algorithm a lot of images.”
The system has to understand what a defect looks like, but unlike a human, it needs a lot of examples to fully comprehend what it’s being asked to look for and recognize.
“So, let’s say we want it to look for a crack on a part,” Sarkar adds. “You have to give it thousands of images, not only of the cracked part, but you have to also label or annotate that there is a crack in those images. That is a very labour-intensive task, unfortunately.”
To make the process less time-consuming, companies are working on solutions that will automate the learning process, reduce the need for human intervention, and therefore cut the costs associated with incorporating machine vision into a manufacturing facility.
For example, Siemens’ new SynthAI is a technology that generates thousands of annotated computer-generated synthetic images and then employs them to train the system on what it needs to look for.
Dave Wilson, vice-president of sales at Siemens Digital Industry Software for Canada explains, “The basis of SynthAI is to train the machine learning model by feeding it those images. So in effect, we’re trying to empower the customer. Whether they have a data science staff or not, those skill sets can still be contracted in. But when it comes to the actual teaching of the model, and feeding it all of that data, this solution helps take some of the complexity and manual tasks out of the equation.”
Zebra Technologies recently announced the acquisition of Matrox Imaging, which Donato Montanari says will allow Zebra to make machine vision more accessible and more affordable for manufacturers thanks to Matrox Imaging’s focus on ease-of-use and AI.
Montanari explains how AI is making machine vision more affordable to implement. “All machine vision software is something called ‘rule-based,’ which means a programmer has to write the rules of the defects you’re looking for,” he explains. “That’s why it’s so expensive. You need a programmer who spends, hours, if not days, in your production line, setting up the system.
“With the introduction of artificial intelligence, it’s going to be very different. The camera will be shown components with defects, and it will learn what a defect is. Nobody has to code anything. This will allow discrete manufacturers to be able to deploy the solution virtually by themselves, which would significantly lower the total ownership cost. It would also allow them to really leverage this technology, by using it not just at the end of the production line, but at different points. If we make it cheap enough, you can use it to look at work-in-progress.”
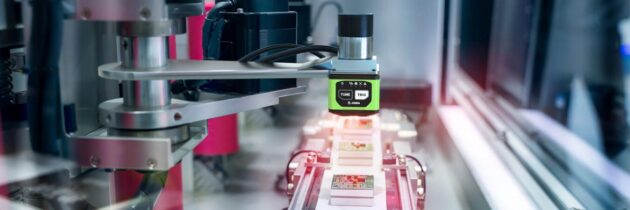
An intelligent machine needs a lot of examples in order to fully comprehend what it’s being asked to look for and recognize. (PHOTO: Zebra Technologies)
Tasks and applications
The potential applications of machine vision seem almost limitless. “There are tons of examples of use cases,” Siemens’ Sarkar says. “Think of human beings and what they’re watching or keeping an eye on. In all these cases, the eyes are simply being replaced by cameras.”
Applications include looking for defects in parts, tracking lot numbers, sorting SKUs, sorting parts, monitoring employees to make sure they’re wearing the proper protective gear, or even whether an employee is operating equipment they’re not trained on, etc. If a human eye can see it, monitor it, track it or record it, then machine vision can probably do the job faster, more accurately, and around the clock, without taking a break.
Sarkar adds that infrared cameras can also be employed, which means the system can be taught to look for hotspots that might mean there’s a problem with a part or a component before it’s shipped.
A closer look
Whether machine vision automation is the right fit for an organization is a question each manufacturer must answer for themselves. However, with the benefits this technology can offer, it’s certainly worth a closer look, especially if costs come down.
“With cost pressures today, automation represents the biggest opportunity to minimize the labour inputs and control those variable costs,” concludes Dave Wilson from Siemens. “Machine vision technologies are one of a plethora of advanced technologies that manufacturers should be exploring as part of their digital transformation endeavours to identify whether or not there are use cases that could drive value for them.”
As costs come down, thanks in part to advancements in AI, we’re likely to see machine vision employed in a growing number of applications. “This is not a new technology,” stresses Zebra’s Montanari. “People in the quality control and manufacturing businesses understand the value of machine vision. But sometimes it’s not feasible for them, because it’s too complex, and complexity translates into cost and time. So, the easier machine vision becomes, the more we can expect it to become a predominant technology.”
Advertisement
- Canada’s inflation not as bad as some of its global counterparts
- Supply chain solutions for the future